brAInfood: How to explain the decisions made by an AI system?
Date of publication: December 2020 /
WHAT IS THIS BRAINFOOD ABOUT?
How can you know if the decision made by an AI system is correct, honest and no error occured in the system? This is further investigated at 'Explainable AI'.
In this brAInfood, we focus on the explainability of AI systems. What does explainability mean? How can AI systems be explained? What obligations do you have as an AI developer towards your users when they ask about the explainability of your AI system? Which domains already focus on explainable AI systems?
WHY SHOULD I READ THIS?
Are you looking for more information about explainability of AI systems? Then, this brAInfood will be interesting for you. We hope to give you more insight into the importance and usefulness of explainable AI systems, why explainability is an added value for your AI system and what rights users have with regard to the explainability of AI systems. Based on some application examples, we stress the importance and need for 'explainable AI'.
WHAT CAN I FIND OUT WHEN READING THIS?
This brAInfood from the Knowledge Centre Data & Society includes:
- A short explanation on explainability: what does it mean?
- More information on white box, black box and grey box: how can explainability be applied?
- A short summary of how you, as a user, can gain insight into the made decisions.
- For the prurpose of illustration, 4 examples of domains in which 'explainable AI' is very important.
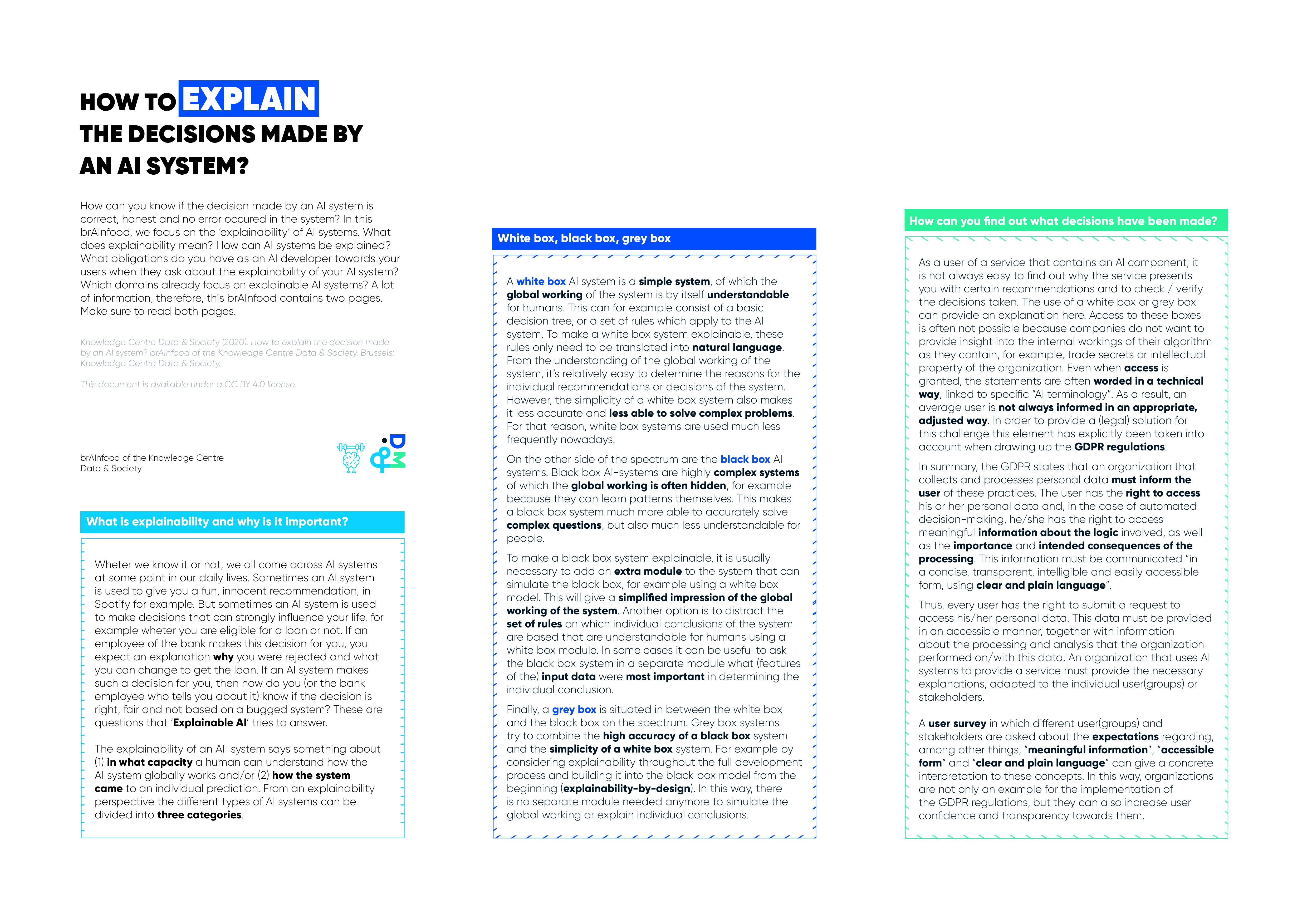

DOWNLOADS
With brAInfood, the Knowledge Centre Data & Society wants to
provide easily accessible information about artificial intelligence.
brAInfood is available under a CC BY 4.0 license, which means that you can reuse and remix this material, provided that you credit us as the source.